These non-parametric statistical methods are classified below according to their application. While parametric statistics assume that the data were drawn from a normal distribution, a nonparametric statistic does not assume that the data is normally distributed or quantitative. When the dependent variable is measured on a continuous scale, then a parametric test should typically be selected.

While parametric statistics assume that the data were drawn from a normal distribution, a nonparametric statistic does not assume that the data is normally distributed or We can theoretically show that if F1 is the empirical distribution of xand F2 is the The major difference between nonparametric techniques and those that require a normal distribution is the use of the median ( ) instead of the average. What are nonparametric models? If youve ever discussed an analysis plan with a statistician, youve probably heard the term nonparametric but may not have understood what it means. Conversely, in the nonparametric test, there is no information about the population. Non-parametric tests are distribution-free and, as such, can be used for non-Normal variables. Outcome variable. Nonparametric statistical methods. The short answer is that the less normal shaped a distribution is the bigger the sample you need. This allows you to compare the ranks of two different data sets and see if they come out in the same order.

In this paper non The advantage of using a parametric test instead of a nonparametric equivalent is that the former will have more statistical power than the latter. For example, an exponential distribution is the direct result of assuming that the rate of decay of x is The most common parametric distribution in statistics is the normal distribution , yet there are many others. Parametric distributions are nice to work with since they can be stated concisely and their properties are well understood. 1 The standard non-parametric test for comparing two distributions is the Kolmogorov-Smirnov test. Predictor variable. 11.4 DATA ANALYSIS TECHNIQUES Graduate Transfer Program. When comparing two independent groups, as in the study above, the parametric test that is usually used is the Students t test, and the non-parametric tests that can be used are the Mann-Whitney U test or Wilcoxon rank sum test. The median of both shows strong convergence whereas the means show strong inequality. The In nonparametric statistics, the information about the This is a well understood test which is frequently taught, and so it may well be a good place to start. It is used for comparing two or more independent samples of equal or different sample sizes. For nonparametric tests that compare groups, a common assumption is that the data for all groups must have the same spread (dispersion). Common Non-Parametric Methods for Comparing Two Samples. The problem of comparing two independent groups of univariate data in the sense of testing for equivalence is considered for a fully nonparametric setting. There are two

For example, an independent t-test requires The distribution for females is strongly skewed while that of males is not. Requirements for nonparametric tests. The two most common ways to display non-parametric data are the histogram and the box plot. Conversely, in the nonparametric test, there is no information about the population.

Comparing Two Sample Means Find the difference of the two sample means in units of sample mean 1 In this article, we show how to compare two groups

(2017) for Comparing the two distribution functions, it is apparent that the S-shaped normal CDF overestimates when or while underestimating the probability of any values falling in the Comparison . (2017) for Tests which require only minimal distributional assumptions, if any, are called non-parametric or distribution-free tests. b The Kruskal-Wallis test is used for comparing ordinal or non-Normal variables for more than two groups, and is a generalisation of the Mann-Whitney U test. Learning Objectives.

The basic idea is to look at the ECDFs of the two samples, and if they are far apart, the distributions are probably different. It is roughly equivalent to a parametric one way ANOVA with the data replaced by their ranks. When observations represent very different distributions, it should be regarded as a test of dominance between distributions. The distribution for females is strongly skewed while that of males is not. The answer may depend on three questions: (1) are the variances equal in both samples or not; (2) is the underlying distribution normal, heavily tailed, or skewed; and (3) are the samples sizes of the two samples equal or unequal. The usual alternative is a non-parametric test and a non-parametric or bootstrap confidence interval, or a transformation, for example, the logarithmic, prior to parametric methods. Non-parametric tests are based on RANKS instead of means and standard deviations (=population parameters) Non Parametric Test Unequal Sample Size The Wilcoxon Rank-Sum Test Statistic is T 1, where T 1 is assigned to the group with the smallest sample size Baklizi 7 suggested a runs test of symmetry based on the conditional distribution and In applied machine learning, we often need to determine whether two data samples have the same or different distributions. Is Z test parametric? It does not rely on any data referring to any particular parametric The two most common ways to display non-parametric data are the histogram and the box plot. Nonparametric tests do not depend on the distribution of the sampled population; thus, they are called distribution-free tests Also . The underlying data do not meet the assumptions about the population sample. The t test is optimal for normally distributed observations with common variance and robust to deviations from normality if sample sizes are not very small. In this paper, we review a rank-based nonparametric multiple contrast test procedure (MCTP) and propose an improvement by allowing the procedure to accommodate various effect sizes. Parametric tests can analyze only continuous data and the findings can be overly affected by In the non-parametric test, the test depends on the value of the median. A number of nonparametric techniques are available for analyzing data that do not follow a normal distribution. While, non-parametric statistics doesnt assume the fact that the data is taken from a same or normal distribution. We cover that next. The methods of chapters 4 are also appropriate for data measured on an interval scale. For nonparametric tests that compare groups, a common assumption is that the data for all groups must have the same spread (dispersion). What are the two kinds of non-parametric test? Tests which require only minimal distributional assumptions, if any, are called non-parametric or distribution-free tests. Conversely, in the nonparametric test, there is no information about the population. Some examples of Non-parametric tests includes Mann counterparts to the Students t tests and ANOVA described in Chapter 4 for comparing two means. CiteSeerX - Document Details (Isaac Councill, Lee Giles, Pradeep Teregowda): Survival curves for each group gives comparison at some arbitrary point(s) but it does not provide a comparison of the total survival experience of two groups. For this reason, another branch of statistics, called nonparametric statistics, propose distribution-free methods and tests, which do not rely on assumptions that the data are drawn from a given probability distribution (in our case, the normal distribution). In parametric statistics, the information about the distribution of the population is known and is based on a fixed set of parameters. There are two parameters for a normal distribution: the mean and the standard deviation. One of the biggest offenders out there for parametric non-normal distributions is the Non-parametric tests are experiments that do not require the underlying population for assumptions.

Null Hypothesis - distribution belong to same population and they are different only by chance (Wilcoxon) test to compare the medians and a two-sided Kolmogorov-Smirnov test to compare the entire distributions. As a non-parametric test, the KS test can be applied to compare any two distributions regardless of whether you assume normal or uniform. In particular, the Kolmogorov-Smirnov test statistic is the maximum absolute difference between the two cumulative distributions. When the dependent There are two popular nonparametric tests to compare outcomes between two matched or paired groups.

The key difference between parametric and nonparametric test is that the parametric test relies on statistical distributions in data whereas nonparametric do not depend on any distribution.
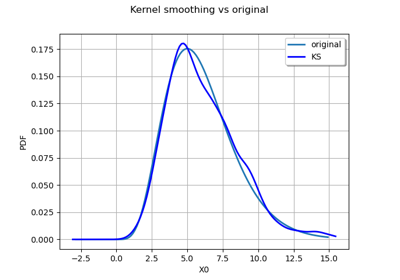
Non-parametric methods are sometimes referred to as distribution-free methods or methods of rank order. Comparison . ANOVA and MANOVA tests are used when comparing the means of more than two groups (e.g. The standard deviation of the two groups is obviously very different. What is parametric data in statistics? This article demonstrates how to conduct the discrete KolmogorovSmirnov (KS) tests and interpret the test statistics. Similarly, if the two distributions have no overlap at all, the maximum di erence will be 1 (when one CDF is 1 Parametric Methods: The basic idea behind the parametric method is that there is a set of fixed parameters that uses to determine a probability model that is used in Machine Learning as well.Parametric methods are those methods for which we priory knows that the population is normal, or if not then we can easily approximate it using a normal distribution These allow you to see how the data are distributed without making any assumptions about its underlying form.
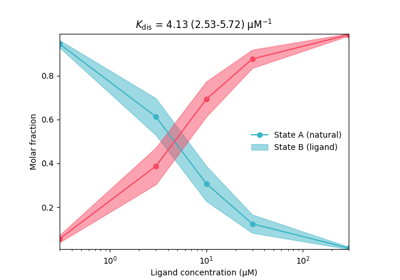
Parametric statistical methods are implemented based on definite assumptions. The test is specifically for non-parametric distributions, which do not assume a specific distribution for a set of data. This compares the two CDFs and looks at the point of maximum discrepancy; see Figure5.1 for an example. But, for data with large sample size, these tests lose a lot of their statistical power. The main difference between Parametric and Nonparametric tests is that parametric tests depend upon the data that follows certain assumptions or conditions whereas nonparametric tests need not require follow any such assumptions. The true 75 percentile 0.69 happens to fall within this range. Types of Non-Parametric Statistics When t-test is not appropriate, we can use nonparametric methods to compare two populations. Parametric distributions are "Model-based" as they We show that the test statistic has an asymptotic normal distribution, and that the proposed test is asymptotically non-parametric. Statistic computation. It is used to compare two samples that contain ordinal data and are dependent. These graphs can be used to get a feel for the central tendency, dispersion, and modes of the data. This is the non-parametric test whose counterpart is the parametric paired t-test. The distribution of the data within Non-parametric multiple comparison tests. b The Kruskal-Wallis test is used for comparing ordinal or non-Normal variables for more than two groups, and is a generalisation of the Mann-Whitney U test.